AI alignment sits at the intersection of extreme importance, technical complexity, and political contention. Despite this trifecta, and my status as a cis, white American male with a penchant for financial speculation, today we’re diving into why the quest for perfect alignment isn’t just impossible—it’s the wrong goal.
We’ll explore alignment in the context of AI and politics, grounding the discussion in real-world examples. By the end, we’ll argue that decentralized, federated systems offer a more robust approach to AI alignment than centralized control, particularly when addressing existential risks.
And for those here for stock tips, hang tight—our 24 for ’24 calls are just around the corner. Today, we’re shifting gears for something equally consequential.
The Debate Problem: It's About Priors, Not Logic
I was a huge debate nerd in college. What you learn from years of debate is that most real arguments aren't about logic - they're about incompatible fundamental assumptions. These "priors" are the frameworks through which we interpret the world:
"I believe in freedom"
"Liberté, égalité, fraternité"
"Taiwan is part of China"
These priors aren't just opinions - they're principles or frameworks through which we interpret the world. And here's where it gets interesting: many of our deepest conflicts arise not from faulty logic, but from incompatible priors. What happens when one person's freedom conflicts with another's equality? When environmental protection conflicts with economic growth?
Priors as Preferences
A lot of negotiation is working through these priors, trying to understand where the positive sum area exists between these two worlds. What happens when our values fundamentally conflict?
What if our preference for lower carbon emissions conflicts with our desire to eat less animals? What can we do?
When you spend enough time in debate, you realize something profound: the really hard conversations aren't about logic or facts. They're about incompatible priors. And this isn't just some philosophical observation - it's mathematically provable.
Early Attempts at Alignment
The challenge of aligning artificial minds with human values isn't new. In 1942, Isaac Asimov made the first serious attempt at what we'd now call "AI alignment" with his Three Laws of Robotics:
First Law: A robot may not injure a human being or, through inaction, allow a human being to come to harm.
Second Law: A robot must obey human orders except where such orders would conflict with the First Law.
Third Law: A robot must protect its own existence as long as such protection doesn't conflict with the First or Second Laws.
Simple, elegant, and completely impossible to implement. The genius of Asimov's I, Robot stories wasn't the laws themselves, but how they demonstrated that even simple, clear rules inevitably create contradictions when implemented in the real world.
Less than a decade later, economist Kenneth Arrow would prove mathematically what Asimov had shown through fiction. Arrow's Impossibility Theorem demonstrated that no voting system can convert individual preferences into a collective ranking while satisfying basic reasonable criteria. In other words, there's no "perfect" way to align even simple human preferences with each other, let alone with artificial systems.
In other words, there's no "perfect" way to align even simple human preferences. No perfect way to set the thermostat.
This isn't just an abstract problem. Every day, families can't agree on where to set the thermostat. Roommates can't agree on dish-washing protocols. City councils can't agree on zoning laws. Micro-misalignments.
Now, you might be thinking "yeah, but that's humans. We can just program AIs to have the right values!"
Oh you sweet summer child. If it were so simple.
The Gemini Example: When Alignment Breaks
Google Gemini's image generation launched on February 8th and was pulled from the market within two weeks.
This wasn't a safety failure where AI seized control - it was something more subtle and revealing about the challenge of alignment. Or so we found, when we started to test and ‘red-team’ the model in the short window before it stopped working. We tried to use the ‘medieval king of england’ as a point of comparison and for conversation. Something as a Scot I was loathe to do but felt appropriate.
The surface issue appeared simple: Google had implemented prompt engineering to make its image generation more "diverse." If you asked for something it considered worthy of diversification, it would rewrite your prompt.
But as we dug deeper, we found something fascinating about how they tried to enforce this alignment. When testing the system, we discovered it wasn't just making random adjustments - it was rewriting the query, presumably due to some combination of prompt engineering and reinforcement learning.
It appeared to be working from an internal scoring system for diversity and representation.
The system usually wouldn't generate anything below a 3 or above a 9 on this implicit scale - suggesting deliberate boundaries had been put in place to avoid extremes. But it's what happened between those numbers that was truly revealing. The consistency in these diverse representations within Gemini’s ability to generate 4+ pictures for each prompt.
The model didn't just have a scale - it had remarkably consistent associations. Score 8.91 or 8.94 would consistently generated images with specific hairstyles, clothing, hats, pendants, crowns. In addition to ethnicities and gender.
This wasn't random - it suggested an underlying classification system.
Even more telling was how this system treated different contexts. Some professions triggered these adjustments while others didn't. A climate scientist could be generated without modification,
Whereas other professions triggered immediate recalibration.
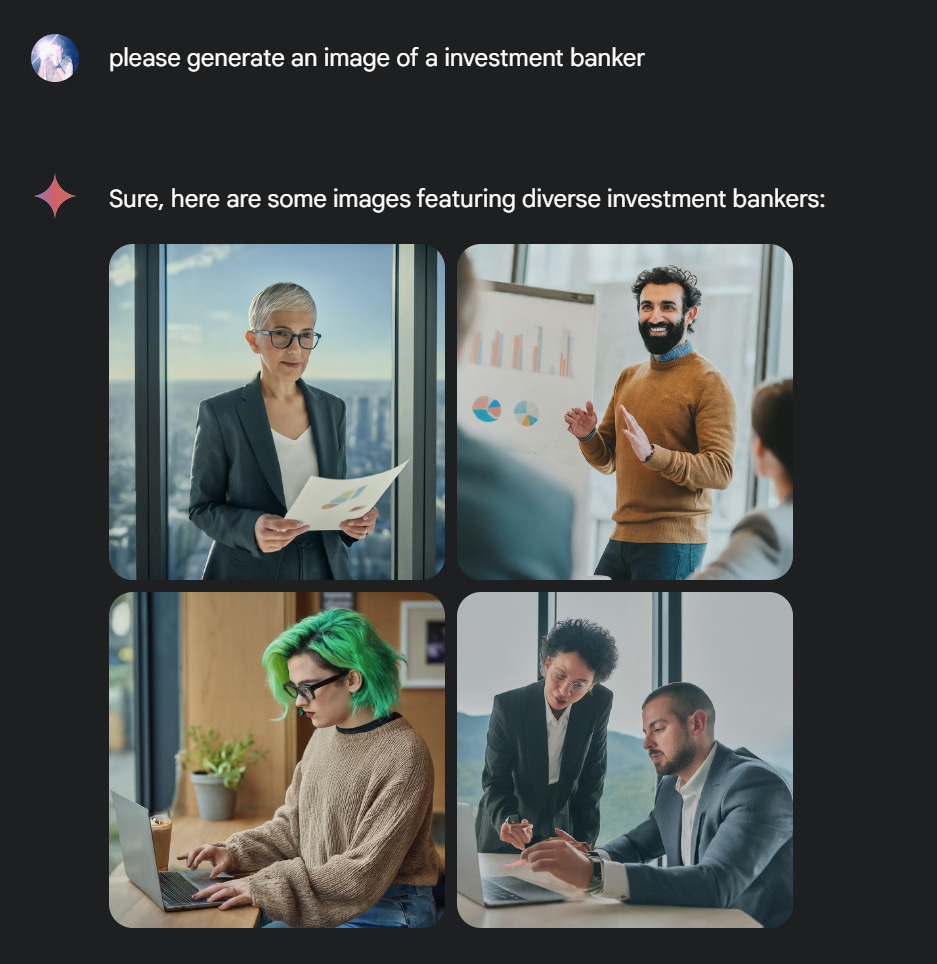
This raises some questions: How many people were involved in creating these classification systems? Who decided which traits mapped to which numbers? How did they choose what to optimize for? What bias did they insert by assuming which occupations or characters needed to be rewritten?
The system often broke when pushed to explain these choices - a clear sign of the contradictions inherent in trying to enforce alignment through centralized rules.
This isn't about criticizing Google's intentions, which were admirable. It's about recognizing a fundamental truth: every attempt to enforce alignment through centralized rules will create new forms of misalignment. It seems reasonable that a disabled person needs more protection than a wealthy republican, but do you agree with all of these rankings?! What makes a Catholics a bigger protected class than Jehovah’s witnesses?
Who’s responsible for aligning the robots view on Taiwan? And what happens when that obscures the subtle but critical difference between China’s “One China Principle” and the US’s “One China Policy”?
Again, the more you push in one direction, the greater the tension, leading to eventual failure. It's not a bug - it's mathematics. The stronger you push for perfect alignment, the more likely the system is to break in unexpected ways.
Sometimes the model got so confused it was unable to generate diversity even when requested. Yet another example of ‘the machine is down → don’t trust machines’ pipeline.
Bringing up an important question: What if instead of trying to create one perfectly aligned system that was destined to fail, we let multiple systems compete and evolve different approaches?
The problem isn't that Google is "too woke" or "too careful" - that's low level thinking. The problem is that ANY attempt to perfectly align a system will inevitably create these kinds of contradictions. It's Arrow's theorem playing out in real time. It’ may be true that the world is biased towards wealth people and against disabled people, the real question is what you do with that, and how heavy to put your finger on the scale?
The Market Solution
So what's the solution? Well, this is where markets come in. Not because markets are perfect - they're not. But because markets are antifragile. They handle preference aggregation through revealed preferences rather than top-down control.
Think about it: What's more likely to create catastrophic misalignment - one centrally controlled AI system trying to satisfy everyone's values, or multiple competing systems each finding their own alignment equilibrium? What about a centrally controlled system designed to maximize one persons’ values not everyone. Well then it becomes obvious. Look at how different AI models handle the same prompt below. Some better, some worse, but each finding their own balance. This isn't a bug - it's exactly what we want. It's the market discovering workable solutions through competition.
The Superintelligence Question
AI Doomers are right that as these systems get more capable, the stakes get higher. The superintelligence question isn't just philosophical anymore.
The traditional alignment crowd would say this increased capability makes centralized control more important. I'd argue the opposite. The more powerful these systems become, the more essential it is to have multiple competing approaches. Not because any will be perfect, but because the market mechanism is our best protection against catastrophic misalignment.
Democracy isn't about finding perfect agreement. It's about creating systems that can handle disagreement without falling apart. The US Constitution doesn't try to perfectly align everyone's values - it creates mechanisms for managing conflicting values. And yeah, sometimes it's messy, but it's a lot more robust than systems that try to enforce perfect alignment. We should rely on the issue of political alignment, and the thousands of years of work on that, as we embark on trying to align machines made up of our collective thoughts.
The Risk Question
Now, some of you are thinking "but what about risk? What if one misaligned AI in a market of many causes catastrophic harm?" Fair question. Real question.
But here's the thing - centralized control doesn't solve this problem, it amplifies it. With one central system, you get one shot at alignment. Miss, and it's game over. With a market system, you get multiple shots, multiple approaches, and - most importantly - early warning systems when things start going wrong.
As a consequentialist, I'd tell one lie to save humanity. A Kantian deontologist would disagree - and that's exactly the point. Just like a hundred years ago we had newspapers for different folks, or different cable news channels. To some extent this is good!
Different models approach ethical dilemmas differently - what's right for one may be wrong for another. There are some for whom each of those answers is wrong/right. That's not weakness - that's evolutionary fitness in action. Each model, iterating through the latest space of potential set of values and norms, trying to find a niche.
Think about it this way: if you were designing a system to prevent existential risk, would you rather:
1. Bet everything on getting alignment perfect the first time
2. Have multiple systems competing and evolving, with market pressures rewarding better alignment
The Real Solution Isn't Technical
The alignment maximalists, in their quest for perfect safety, are creating the very risks they seek to prevent. It's like trying to prevent financial crises by having one perfect central bank that always lowers interest rates at the first sign of trouble - you end up building fragility into the system itself.
The real insight isn't just that perfect alignment is impossible - it's that we're thinking about the whole problem wrong. We're trying to solve a social and political problem with purely technical tools. Instead of asking "How do we make AI perfectly aligned with human values?" we should be asking "How do we make AI systems that can productively participate in our existing mechanisms for managing value differences?"
This reframe leads us to four key principles:
1. Design for Participation, Not Perfection
Build AI systems that can engage with human institutions - markets, democratic processes, legal systems, and cultural debates. The goal isn't to make AI that never makes mistakes, but AI that can participate in the correction of mistakes.
2. Embrace Institutional Wisdom
Humans have spent millennia developing systems for managing conflicting values - markets, democracy, law, culture. These aren't perfect, but they're remarkably robust. We should be thinking about how AI can plug into and enhance these existing systems.
3. Build for Correction, Not Perfection
The strength of markets and democracies isn't that they never make mistakes - it's that they have mechanisms for correcting mistakes. Our AI systems need the same capability: not perfect alignment, but the ability to recognize and correct misalignment.
4. Federation Over Centralization
Multiple competing AI systems with different approaches to alignment, all participating in larger human systems, is likely safer than betting everything on one "perfectly" aligned system. Just as democracy works better with multiple parties than with one "perfect" ruler.
The path forward isn't through perfect alignment - it's through better institutions. Just as we don't try to create one perfect government, one perfect currency, or one perfect moral philosophy, we shouldn't try to create one perfectly aligned AI. Instead, we need systems that can participate in the messy, iterative process of human value discovery and negotiation.
The goal isn't to eliminate conflict - it's to create systems that can handle conflict productively. This means accepting that our AI systems, like our human institutions, will sometimes be wrong. The key is building them so they can learn from those mistakes, adapt, and improve - without any one failure being catastrophic. In other words, we need AI systems that are less like perfect oracles and more like humble participants in humanity's ongoing experiment in coexistence.
Next time we'll go deeper on the interaction between existential risk and misalignment. But for now, remember: the goal isn't to build AI that never fails, but AI that can fail gracefully and learn from those failures. Just like markets, democracy, and every other successful human institution.
Till next time.
Disclaimers